ImmuneScore as a novel RNA-based prognostic signature superior to PD-L1 in advanced non-squamous NSCLC patients receiving chemotherapy combined with immune checkpoint inhibitor therapy
Abstract
Aim: Our study aimed to explore the prognostic predictive potential of a novel RNA-based signature called ImmuneScore in advanced non-squamous NSCLC patients receiving combined immune checkpoint inhibitor (ICI) treatment and chemotherapy.
Methods: RNA-sequencing data of 113 patients screened out from ORIENT-11 trial were retrospectively analyzed. ImmuneScore was calculated by the ESTIMATE algorithm. The association of ImmuneScore with early tumor progression, progression-free survival (PFS), and overall survival (OS) was analyzed using chi-square test, Cox regression test, and log-rank test. Receiver operating characteristic (ROC) curves were generated, with higher values of area under the ROC curves (AUCs) indicating better prediction ability.
Results: ImmuneScore was negatively correlated with early tumor progression rate (4.3% vs. 18.6%, P = 0.013) while positively correlated with PFS (HR = 0.29, 95%CI: 0.16-0.53, P < 0.001) and OS (HR = 0.32,
Conclusion: ImmuneScore serves as a novel RNA-based prognostic signature superior to PD-L1 in advanced non-squamous NSCLC patients receiving chemotherapy combined with ICI therapy. Higher ImmuneScore indicates lower early tumor progression rate, longer PFS, and longer OS.
Keywords
INTRODUCTION
Lung cancer remains the leading cause of cancer-related death worldwide[1] . Broadly, lung cancer is divided into small-cell lung cancer and non-small-cell lung cancer (NSCLC). At present, the chemotherapy and immune checkpoint inhibitor (ICI) combination has emerged as the standard first line of care for advanced NSCLC patients without sensitizing epidermal growth factor receptor (EGFR) or anaplastic lymphoma kinase (ALK) genomic aberrations[2-4]. However, clinically effective biomarkers in this combination chemoimmunotherapy population are still lacking[5,6]. Though Programmed death-ligand 1 (PD-L1) tumor proportion score (TPS) assessed by immunohistochemistry (IHC) serves as a well-recognized biomarker in predicting ICI therapy efficacy, contributing to selecting ideal ICI treatment candidates[7,8], its prognostic value in this combination therapy population remained to be suboptimal[9,10]. To overcome this dilemma, there have been some studies exploiting potential clinical factors like gender, performance status, and concomitant medication administration through meta-analysis[11-13]. In recent years, molecular biomarkers like DNA and RNA signatures developed based on high‐throughput sequencing data have raised concerns[14-16], but studies remain insufficient.
Yoshihara developed the “Estimation of STromal and Immune cells in MAlignant Tumors using Expression data” (ESTIMATE) algorithm, via which the level of tumor-infiltrating immune cells could be quantified as the immune score (ImmuneScore), based on RNA expression profiles[17]. Its clinical application value has not been elucidated yet. The current study aimed to investigate the prognostic value of this RNA-based signature in advanced non-squamous NSCLC patients receiving chemotherapy combined with ICI therapy through a retrospective study containing 113 patients. Results indicated that ImmuneScore was significantly positively associated with prognosis in this population, with predictive ability superior to PD-L1.
METHODS
Inclusion and exclusion criteria
Patients included in our current study were screened out from those participating in ORIENT-11 trial. The selection process is depicted as a flowchart in Figure 1. As we know, ORIENT-11 trial is a double-blind, multicenter, phase 3 study conducted in China, which enrolled 397 advanced non-squamous NSCLC patients from August 23, 2018 to July 30, 2019 to randomly receive either combination chemoimmunotherapy (sintilimab immunotherapy plus platinum-pemetrexed standard chemotherapy) or platinum-pemetrexed chemotherapy (ClinicalTrials.gov: NCT03607539). More specific details of the study design were provided in our published article[18] and thus did not belabor here. Ethical permission and patient consent were obtained. Among the total 397 patients in ORIENT-11 trial, 171 had baseline Formalin-Fixed Paraffin-Embedded (FFPE) tumor samples that met the criteria for RNA sequencing. Of these, 113 patients from the sintilimab plus standard chemotherapy group were finally included in our current study and retrospectively analyzed. Clinical data like age, gender, and smoking history had been collected before the initial treatment.
Calculation of PD-L1 TPS
PD-L1 TPS, defined as the percentage of tumor cells with partial or complete membrane staining of PD-L1, was evaluated on baseline tumor tissues using PD-L1 immunohistochemical 22C3 pharmDx assay (Agilent Technologies). Samples were considered to be PD-L1-positive if PD-L1 TPS was ≥ 1%.
RNA sequencing and ImmuneScore calculation
Baseline FFPE tissues were obtained before treatment by bronchoscopic biopsy or percutaneous needle biopsy. RNA was isolated from 15-20 tissue slides (10-15 μm thick, about 0.25 cm2). cDNA library was synthesized with the NEBNext Ultra II Directional RNA Library Prep Kit for Illumina (NEB, USA). Subsequent RNA sequencing was conducted on the Illumina NovaSeq 6000 platform, and reads were mapped to human genome version 38 (GRCh38). The raw reads and counts were normalized to the number of transcripts per kilobase million. Based on gene expression profiles, the ImmuneScore for each of the 113 patients was calculated using ESTIMATE analysis with the “ESTIMATE” R package provided by
Evaluation of clinical efficacy and study outcome
Treatment efficacy evaluation data had been generated during ORIENT-11 trial by a blinded independent radiographic review committee (BIRRC), based on Response Evaluation Criteria in Solid Tumors (RECIST) 1.1 criteria. Efficacy was classified as complete response (CR), partial response (PR), stable disease (SD), or progressive disease (PD). Patients’ survival status was followed up by regular phone calls by clinical research coordinators. Progression-free survival (PFS) was defined as the time from treatment assignment to disease progression or death from any cause, and overall survival (OS) was defined as the time from treatment assignment to death from any cause. The PFS data were last updated on May 15, 2020 and the OS data were last updated on January 15, 2021. Details were described in our previous published articles[18,19]. PD that occurred within 6 weeks was defined as “early tumor progression” here; otherwise, it was defined as disease control. PFS and OS were set to be primary endpoint, and early disease control served as the secondary outcome
Evaluation of predictive accuracy
Receiver operating characteristic (ROC) analysis was performed to evaluate the predictive efficacy of variables, with larger area under the curve (AUC) values indicating better predictive accuracy. For early tumor progression prediction, ROC curves were generated with the “pROC” R package, which is used for analyzing dichotomic data. For PFS and OS prediction, ROC curves were generated via the “TimeROC” R package, which is specific for survival data. The optimal cut-off point of ImmuneScore was determined considering the highest Youden Index (sensitivity + specificity - 1).
Nomogram construction
Nomograms for PFS and OS prediction were established via the “rms” R package, based on significant prognostic variables identified by multivariate COX regression analysis. The total points for each patient were calculated as the sum of the points for each variable. Calibration curves and decision curves were performed to assess the calibration performance and clinical utility of nomograms.
Gene set enrichment analysis of the high- and low-ImmuneScore groups
Gene set enrichment analysis (GSEA) was carried out with the “fgsea” R package to identify the significant Kyoto Encyclopedia of Genes and Genomes (KEGG) signaling pathways involved in the high- versus low- ImmuneScore group, utilizing “c2.cp.kegg.v7.1.symbols.gmt” downloaded from Molecular Signatures Database (MSigDB) as the reference gene set. Adjusting P-value < 0.05 was regarded as statistically significant.
Statistical analysis
Continuous variables were described as median (range/interquartile range) and were compared using Student’s independent samples t-test. Qualitative variables were presented as frequencies and percentages and were examined with the chi-square test. Before statistical tests, missing values including three tumor
RESULTS
Demographic and clinical characteristics of the patient cohort
As shown in Figure 1, after excluding patients who did not meet the inclusion criteria, 113 non-squamous NSCLC patients receiving chemotherapy combined with the anti-PD-1 antibody sintilimab were retained and retrospectively analyzed. The demographic and clinical characteristics of these patients are shown in Table 1. Patients were diagnosed as stage IIIB to IV (in accordance with the eighth edition of the AJCC TNM classification) without EGFR and ALK mutation. The ESTIMATE algorithm was used to determine the ImmuneScore for each patient. Their ImmuneScores were distributed between -573 and 3,141
Demographic and clinical characteristics of the study cohort
Variables | Patients (N = 113) |
Age (y) | 61 (30-74) |
Gender | |
Male | 87 (77.0%) |
Female | 26 (23.0%) |
ECOG | |
0 | 29 (25.7%) |
1 | 84 (74.3%) |
Smoking status | |
Previous/Current smoker | 42 (37.2%) |
Never | 71 (62.8%) |
PD-L1 TPS | |
< 1% | 31 (27.4%) |
≥ 1% | 82 (72.6%) |
T-stage | |
T1 | 19 (16.8%) |
T2 | 30 (26.5%) |
T3 | 19 (16.8%) |
T4 | 42 (37.2%) |
Tx | 3 (2.7%) |
N-stage | |
N0 | 7 (6.2%) |
N1 | 2 (1.8%) |
N2 | 30 (26.5%) |
N3 | 70 (61.9%) |
Nx | 4 (3.5%) |
M-stage | |
M0 | 9 (8.0%) |
M1 | 104 (92.0%) |
TNM stage | |
IIIB | 7 (6.2%) |
IIIC | 2 (1.8%) |
IV | 104 (92.0%) |
Initial response | |
PR | 35 (31.0%) |
SD | 67 (59.3%) |
PD | 11 (9.7%) |
PFS (months) | 9 (1-14) |
OS (months) | 24(3-37) |
Survival endpoint | |
Dead | 53 (42.5%) |
Alive | 60 (57.5%) |
ImmuneScore as a predictor of early tumor progression
We classified patients into the PD-L1-positive group (82/113) or PD-L1-negative group (31/113) according to the 1% threshold. In the PD-L1-positive group, 76 patients (92.7%) achieved disease control (SD + PR): 47 patients (57.3%) exhibited SD and 29 patients (35.4%) exhibited PR. In the PD-L1-negative group, 26 patients (83.9%) achieved disease control: 20 exhibited SD (64.5%) and 6 patients (19.4%) exhibited PR. Early tumor progression occurred in 6 patients (7.3%) and 5 patients (16.1%) in PD-L1 positive and negative groups, respectively, without significant difference (P = 0.159) [Figure 2A]. ROC analysis was utilized to evaluate the accuracy of PD-L1 in predicting early tumor progression; the AUC value (0.64,
Figure 2. ImmuneScore as a predictor of early disease progression in patients receiving ICI therapy combined with chemotherapy. (A) PD-L1 TPS could not effectively predict early tumor progression; (B) ROC curve and corresponding AUC for PD-L1 TPS in predicting early disease progression; (C) ImmuneScore could predict early tumor progression; (D) ROC curve and corresponding AUC for ImmuneScore in predicting early disease progression. ICI: Immune checkpoint inhibitor; PD-L1 TPS: programmed cell death 1 ligand 1 tumor proportion score; ROC: receiver operating characteristic curve; AUC: area under the ROC curve; PD: progressive disease; PR: partial response; SD: stable disease.
ImmuneScore 1,060 corresponded to the highest Youden Index and thereby was set as the optimal cut-off point in predicting early tumor progression. According to this threshold,70 patients were assigned to the high-ImmuneScore group and the remaining 43 patients to the low-ImmuneScore group. ImmuneScores were significantly different between high and low-ImmuneScore groups, with median values of 1,867 (interquartile range: 1,400-2,170) and 505 (interquartile range: 115-928), respectively (P < 0.001). As shown in Figure 2C, in the high-ImmuneScore group, disease control was achieved in 67 patients, with 42 demonstrating SD (60.0%) and 25 demonstrating PR (35.7%). In the low-ImmuneScore group, disease control was achieved in 35 patients, with 10 demonstrating PR (23.3%) and 25 demonstrating SD (58.1%). Early tumor progression rate was significantly lower in high-ImmuneScore group than in low-ImmuneScore group [3 (4.3%) vs. 8 (18.6%), P = 0.013]. ImmuneScore demonstrated a better performance than PD-L1 (AUC = 0.68, 95%CI: 0.58-0.76, P = 0.021) [Figure 2D].
ImmuneScore as an independent prognostic signature of PFS
As shown in Figure 3A, ImmuneScore was an effective predictor for median PFS, with predictive accuracy (AUC = 0.67, 95%CI: 0.53-0.81) superior to that of PD-L1 TPS (AUC = 0.58, 95%CI: 0.44-0.72). As determined by the highest Youden Index, 1,060 was considered as the optimal cut-off point of ImmuneScore in predicting PFS.
Figure 3. ImmuneScore as a prognostic indicator of PFS in patients with non-squamous NSCLC receiving ICI therapy combined with chemotherapy. (A) ImmuneScore could predict median PFS, with higher AUC than that of PD-L1 TPS; (B) Patients with high ImmuneScore had longer PFS than those with low ImmuneScore; (C) Higher ImmuneScore indicated longer PFS in PD-L1 TPS ≥ 1% and PD-L1 TPS < 1% subgroups; (D) Higher ImmuneScore indicated longer PFS in PD-L1 TPS ≥ 50% and PD-L1 TPS < 50% subgroups. NSCLC: Non-squamous cell lung cancer; ICI: immune checkpoint inhibitor; PFS: progression-free survival; PD-L1 TPS: programmed cell death 1 ligand 1 tumor proportion score; AUC: area under the ROC curve; IS: ImmuneScore; HR: hazard ratio.
As shown in Table 2, univariate COX regression revealed that high ImmuneScore was significantly associated with longer PFS (HR = 0.30, 95%CI: 0.17-0.54, P < 0.001). In addition, age (HR = 2.14,
Results of univariate and multivariate COX regression analysis of variables for PFS prediction
Variables | Univariate analysis | Multivariate analysis | |||
HR (95%CI) | P-value | HR (95%CI) | P-value | ||
Age (y) | |||||
< 65 | Ref. | Ref. | |||
≥ 65 | 2.14 (1.20-3.80) | 0.010* | 2.21 (1.23-3.95) | < 0.001* | |
Gender | |||||
Male | Ref. | - | |||
Female | 0.67 (0.33-1.36) | 0.271 | |||
ECOG | |||||
0 | Ref. | ||||
1 | 1.50 (0.74-3.01) | 0.259 | |||
Smoking status | |||||
Never | Ref. | - | |||
Previous/Current smoker | 1.10 (0.61-2.00) | 0.758 | |||
PD-L1 TPS | 0.074 | ||||
< 1% | Ref. | - | |||
≥ 1% | 0.59 (0.33-1.05) | ||||
T-stage, per stage | 1.65 (1.25-2.17) | < 0.001* | 1.65 (1.25-2.19) | < 0.001* | |
N-stage, per stage | 1.04 (0.74-1.47) | 0.804 | |||
M-stage M0 | Ref. | ||||
M1 | 3.12 (0.75-12.90) | 0.117 | |||
ImmuneScore | |||||
Low | Ref. | - | Ref. | ||
High | 0.30 (0.17-0.54) | < 0.001* | 0.29 (0.16-0.53) | < 0.001* |
Kaplan-Meier survival analysis was conducted to compare PFS between the high- and low-ImmuneScore groups. 48 patients experienced progression during the follow-up, while 65 patients without progression were censored. The high-ImmuneScore group had longer median PFS than the low-ImmuneScore group (7.2 vs. 11.4 months; HR = 0.25, 95%CI: 0.14-0.48, P < 0.001) [Figure 3B]. Similar results were obtained in subgroup analysis based on PD-L1 TPS expression (PD-L1 ≥ 1%: HR = 0.33, 95%CI: 0.14-0.80, P = 0.014; PD-L1 < 1%: HR = 0.25, 95%CI: 0.10-0.66, P = 0.005; PD-L1 ≥ 50%: HR = 0.30,
A nomogram was constructed by incorporating three statistically significant variables identified in the multivariate COX regression analysis mentioned above, i.e., age, tumor T-stage and ImmuneScore [Figure 4A]. The nomogram exhibited good predictive performance in predicting 4-month PFS rate
Figure 4. Construction of prognostic nomogram models. (A) Prognostic nomogram model construction for PFS prediction; (B) ROC curves and corresponding AUCs for variables in predicting PFS; (C) Prognostic nomogram model construction for OS prediction; (D) ROC curves and corresponding AUCs for variables in predicting OS. NSCLC: Non-squamous cell lung cancer; ICI: immune checkpoint inhibitor; PFS: progression-free survival; OS: overall survival.
ImmuneScore as an independent prognostic signature of OS
As shown in Figure 5A, ImmuneScore was an effective predictor for median OS, with predictive accuracy (AUC = 0.73, 95%CI: 0.61-0.85) higher than that of PD-L1 TPS (AUC = 0.63, 95%CI: 0.50-0.76). 1,005 was set as the optimal ImmuneScore cut-off point in predicting OS with the highest Youden index.
Figure 5. ImmuneScore as a prognostic indicator of OS in patients with non-squamous NSCLC receiving ICI therapy combined with chemotherapy. (A) ImmuneScore could predict median OS, with higher AUC than that of PD-L1 TPS; (B) Patients with high ImmuneScore had longer OS; (C) Higher ImmuneScore indicated longer OS in PD-L1 TPS ≥ 1% and PD-L1 TPS < 1% subgroups; (D) Higher ImmuneScore indicated longer OS in PD-L1 TPS ≥ 50% and PD-L1 TPS < 50% subgroups. NSCLC: Non-small cell lung cancer; ICI: immune checkpoint inhibitor; OS: overall survival; PD-L1 TPS: programmed cell death 1 ligand 1 tumor proportion score; AUC: area under the ROC curve; IS: ImmuneScore; HR: hazard ratio.
As shown in Table 3, univariate COX regression revealed that high ImmuneScore was significantly associated with longer OS (HR = 0.28, 95%CI: 0.16-0.48, P < 0.001). Additionally, gender (HR = 0.46, 95%CI: 0.21-0.98, P = 0.044), tumor T-stage (HR = 1.31, 95%CI: 1.01-1.69, P = 0.036), and PD-L1 TPS (HR = 0.56, 95%CI: 0.32-0.99, P = 0.045) were also significant. After adjusting for these three variables in multivariate COX regression, ImmuneScore remained statistically significant (HR = 0.32, 95%CI: 0.18-0.58, P < 0.001).
Univariate and multivariate COX regression analysis of variables for OS prediction
Variables | Univariate analysis | Multivariate analysis | ||
HR (95%CI) | P-value | HR (95%CI) | P-value | |
Age (y) | ||||
< 65 | Ref. | |||
≥ 65 | 1.68 (0.97-2.89) | 0.064 | ||
Gender | ||||
Male | Ref. | - | Ref. | - |
Female | 0.46 (0.21-0.98) | 0.044* | 0.35 (0.15-0.78) | 0.010* |
ECOG | ||||
0 | Ref. | - | ||
1 | 1.75 (0.85-3.60) | 0.126 | ||
Smoking status | ||||
Never | Ref. | - | ||
Previous/Current smoker | 1.48 (0.83-2.64) | 0.187 | ||
PD-L1 TPS | ||||
< 1% | Ref. | - | Ref. | |
≥ 1% | 0.56 (0.32-0.99) | 0.045* | 0.79 (0.43-1.47) | 0.455 |
T-stage, per stage | 1.31 (1.01-1.69) | 0.036* | 1.43 (1.09-1.87) | 0.009* |
N-stage, per stage | 0.97 (0.71-1.33) | 0.869 | ||
M-stage | ||||
M0 | Ref. | |||
M1 | 7.14 (0.98-51.81) | 0.052 | ||
ImmuneScore | ||||
Low | Ref. | Ref. | ||
High | 0.28 (0.16-0.48) | < 0.001* | 0.32 (0.18-0.58) | < 0.001* |
Kaplan-Meier survival analysis of OS was conducted. 53 patients died during the follow-up, while 60 were censored (34 alive and 26 lost to follow-up). Kaplan-Meier survival analysis revealed that the high ImmuneScore group had a longer median OS than the low ImmuneScore group
A nomogram was constructed by incorporating three statistically significant variables identified in multivariate COX regression analysis, that is, gender, tumor T-stage, and ImmuneScore [Figure 4C]. The nomogram exhibited good ability in predicting 6-month OS rate (AUC = 0.82, 95%CI: 0.68-0.95), 12-month OS rate (AUC = 0.74, 95%CI: 0.62-0.86), and 24-month OS rate (AUC = 0.84, 95%CI: 0.75-0.93) [Figure 4D]. The calibration curve and decision curve exhibited good performance
Enrichment of immune response-related pathways in patients with high ImmuneScores
As shown in Figure 6, several immune response-related pathways were significantly enriched in the high-ImmuneScore group, such as KEGG_hematopoietic_cell_lineage, KEGG_cytokine_cytokine_receptor_interaction, and KEGG_T_cell_ receptor_ signaling_ pathway. This suggested that compared with the low-ImmuneScore group, the high-ImmuneScore group had a more active antitumor immune response.
DISCUSSION
Although PD-L1 TPS serves as the most commonly used biomarker in predicting ICI therapy efficacy currently[20], our present study revealed that PD-L1 TPS neither effectively predicts early tumor progression nor PFS in the combination chemoimmunotherapy population. With regard to OS, PD-L1 TPS demonstrated prognostic value in univariate COX regression, but its significance was lost after adjustment in the multivariate analysis. In agreement with our findings, previous studies found that the predictive power of PD-L1 is inadequate for patients receiving ICI therapy combined with chemotherapy[21,22]. Chemotherapy alters the tumor immune microenvironment, and combined immunotherapy and chemotherapy may have a synergistic effect[23,24]. We hence consider that in patients receiving combination therapy, PD-L1 is insufficient to reflect their antitumor immunity level, which may interpret its limited prognostic value in this population.
Some multigene RNA signature-based assays have been used to predict the prognosis of malignancies and assist in treatment decision-making. For instance, the 70-gene signature is used to aid treatment decisions in early-stage breast cancer[25]. However, as far as we know, no RNA-based signature has been clinically applied in the field of lung cancer yet. In our current study, the ImmuneScore for each patient was calculated based on total RNA sequencing data, via the ESTIMATE algorithm proposed by Yoshihara et al. Briefly, ImmuneScore is calculated as the enrichment score of a gene set containing 141 immune-related genes, on the basis of the ssGSEA algorithm[17]. Our results revealed that ImmuneScore had prognostic value in advanced non-squamous NSCLC patients receiving chemotherapy combined with ICI therapy, with higher AUCs than that of PD-L1, indicating its relatively superior predictive ability. Specifically, patients with higher ImmuneScores had lower early tumor progression rates, longer PFS, and longer OS than those with lower ImmuneScores. The available evidence suggests that the tumor immune microenvironment is an important determinant of sensitivity to ICI treatment[26,27]. We consider that ImmuneScore seems to be a biomarker assisting in distinguishing so-called “immune-hot tumors” or “immune-cold tumors”[28]. According to the present GSEA results, patients with high ImmuneScore values are likely to have “immune-hot tumors”, which are characterized by active antitumor immune response, high level of immune infiltration, and sensitivity to immunotherapy. In contrast, those with low ImmuneScore values are likely to have “immune-cold tumors”, which are characterized by inactive antitumor response, low level of immune infiltration, and insensitivity to immunotherapy. Additionally, in considering that the current RNA-based signatures are mostly based on absolute RNA expression levels and have limited reproducibility across platforms[29], ImmuneScore may possess specific advantages, since ImmuneScore is calculated by the ssGSEA algorithm, which is based on the rank order and empirical cumulative distribution functions of 141 targeted genes, rather than their absolute expression levels[17].
Based upon our findings, we suggest ImmuneScore be routinely detected in advanced NSCLC patients without sensitive mutation so as to guide clinical decision-making in the future. We are more inclined to recommend those with low ImmuneScore to receive chemotherapy rather than combination chemoimmunotherapy, since they are more likely to possess immune-cold tumors. In contrast, those with high ImmneScore are more likely to possess immune-hot tumors and thus would be recommended to receive combination chemoimmunotherapy. Multidisciplinary collaboration involving clinicians, pathologists and bioinformatics specialists is essential for the construction of “ImmuneScore platform” and quality control. As an RNA-based signature, the clinical application of ImmuneScore faces some challenges. For one, due to the limited stability of RNA and its susceptibility to degradation, cryopreservation and a shorter transportation time for specimens would be required. Further, despite continuing price reductions, the cost of traditional total RNA sequencing is still relatively high. A desirable alternative may be to adopt “gene panels” like tumor mutation burden (TMB) detection, which may accelerate the clinical application process. More cohorts need to be examined in the future to identify and optimize the suitable “RNA panel”.
Apart from immuneScore, our study also identified several valuable clinical parameters including tumor T-stage, gender, and age. Tumor T-stage is mainly determined by tumor size. Previous studies revealed large tumors to be more immunosuppressive and less responsive to cytotoxic treatments compared to small tumors[30,31]. This fits well with our finding that T-stage was negatively associated with both PFS and OS. As to age, patients ≥ 65 years had shorter PFS time than those < 65 years. It may be due to the immunosuppression tumor phenotypes in elderly patients[32]. Their poor tolerance leading to susceptible to dose reduction or treatment delay may also worsen outcomes[33]. In the case of gender, this study found that female patients obtained longer OS time than their male counterparts, which was consistent with a previous meta-analysis[34]. This may account for the different immune features between males and females determined by genetics and sex hormones[35]. It was found that females possessed more abundant immune cell infiltration and more excellent anti-cancer immunity response than males and thus benefit more from immunotherapy[36]. Indeed, the impact of age or gender on immunotherapy or chemoimmunotherapy outcomes remained controversial, with published studies reaching inconsistent conclusions[32,37,38]. Although our current study supported new evidence, prospective cohort studies and molecular biology studies are still needed to further elucidate it.
Some limitations of this study need to be considered. First, our cohort comprised only Chinese patients with non-squamous NSCLC and only sintilimab was used as the ICI therapy regimen. Therefore, generalizability to populations from other regions of the world, patients with squamous NSCLC, or patients receiving treatment with other immunotherapeutic agents needs to be further confirmed. Second, since TMB was not detected in our samples, we could not include this dynamic immunological marker in multivariate regression COX analysis. Third, since patients receiving ICI monotherapy were not available in this study, we could not verify the prognostic value of ImmuneScore in this population. Finally, due to the retrospective nature, we adopted the patients’ efficacy evaluation data generated by BIRRC, based on RECIST 1.1 criteria rather than re-evaluating it by ourselves based on immune RECIST (iRECIST) criteria, which could not identify the so-called pseudoprogression due to immunotherapy[39]. Previous studies suggested that the pseudoprogression considered by iRECIST was rare in NSCLC, occurring in about
In conclusion, ImmuneScore calculated by the ESTIMATE algorithm serves as a promising RNA-based prognostic signature for advanced non-squamous NSCLC patients receiving chemotherapy combined with ICI therapy, with predictive efficacy superior to that of PD-L1 TPS. Specifically, higher ImmuneScore indicates lower rate of early tumor progression, longer PFS, and longer OS. Further, the nomogram integrating ImmuneScore and clinical parameters, such as tumor T-stage, age, and gender, may serve as a tool for prognostic prediction and screening the dominant population to receive combined ICI treatment and chemotherapy.
DECLARATIONS
Authors’ contributions
Contributed to the conception and design of the study: Zhang L, Lin Z
Contributed to the data acquisition and statistical analysis: Lin Z, Zhan J
All authors wrote and approved the final manuscript.
Availability of data and materials
The data that support our findings in this study are available from the corresponding author upon reasonable request.
Financial support and sponsorship
This work was supported by the Medical Science and Technology Research Fund of Guangdong Province (Grant No. C2018062).
Ethics approval and consent to participate
Given the retrospective nature of our current study and the fact that no personal identifiers were contained, the need for ethical approval and individual informed consent was waived by the Ethics Commission of Sun Yat-sen University Cancer Center. All methods were carried out in accordance with the Declaration of Helsinki.
Consent for publication
Given the retrospective nature of our current study and the fact that no personal identifiers were contained, the need for individual informed consent was waived by the Ethics Commission of Sun Yat-sen University Cancer Center. All methods were carried out in accordance with the Declaration of Helsinki.
Conflicts of interest
All authors declare that there are no conflicts of interest.
Copyright
© The Author(s) 2024.
Supplementary Materials
REFERENCES
1. Hu X, Estecio MR, Chen R, et al. Evolution of DNA methylome from precancerous lesions to invasive lung adenocarcinomas. Nat Commun 2021;12:687.
2. Yan Y, Kumar AB, Finnes H, et al. Combining immune checkpoint inhibitors with conventional cancer therapy. Front Immunol 2018;9:1739.
3. Heinhuis KM, Ros W, Kok M, Steeghs N, Beijnen JH, Schellens JHM. Enhancing antitumor response by combining immune checkpoint inhibitors with chemotherapy in solid tumors. Ann Oncol 2019;30:219-35.
4. Rizzo A. Identifying optimal first-line treatment for advanced non-small cell lung carcinoma with high PD-L1 expression: a matter of debate. Br J Cancer 2022;127:1381-2.
5. Bai R, Lv Z, Xu D, Cui J. Predictive biomarkers for cancer immunotherapy with immune checkpoint inhibitors. Biomark Res 2020;8:34.
6. Lei Y, Li X, Huang Q, Zheng X, Liu M. Progress and challenges of predictive biomarkers for immune checkpoint blockade. Front Oncol 2021;11:617335.
7. Topalian SL, Hodi FS, Brahmer JR, et al. Safety, activity, and immune correlates of anti-PD-1 antibody in cancer. N Engl J Med 2012;366:2443-54.
8. Tang B, Yan X, Sheng X, et al. Safety and clinical activity with an anti-PD-1 antibody JS001 in advanced melanoma or urologic cancer patients. J Hematol Oncol 2019;12:7.
9. Shen X, Zhao B. Efficacy of PD-1 or PD-L1 inhibitors and PD-L1 expression status in cancer: meta-analysis. BMJ 2018;362:k3529.
10. Festino L, Botti G, Lorigan P, et al. Cancer treatment with anti-PD-1/PD-L1 agents: is PD-L1 expression a biomarker for patient selection? Drugs 2016;76:925-45.
11. Rizzo A, Cusmai A, Giovannelli F, et al. Impact of proton pump inhibitors and histamine-2-receptor antagonists on non-small cell lung cancer immunotherapy: a systematic review and meta-analysis. Cancers 2022;14:1404.
12. Santoni M, Rizzo A, Mollica V, et al. The impact of gender on the efficacy of immune checkpoint inhibitors in cancer patients: the MOUSEION-01 study. Crit Rev Oncol Hematol 2022;170:103596.
13. Mollica V, Rizzo A, Marchetti A, et al. The impact of ECOG performance status on efficacy of immunotherapy and immune-based combinations in cancer patients: the MOUSEION-06 study. Clin Exp Med 2023;23:5039-49.
14. Jardim DL, Goodman A, de Melo Gagliato D, Kurzrock R. The challenges of tumor mutational burden as an immunotherapy biomarker. Cancer Cell 2021;39:154-73.
15. Strickler JH, Hanks BA, Khasraw M. Tumor mutational burden as a predictor of immunotherapy response: is more always better? Clin Cancer Res 2021;27:1236-41.
16. Zeng TM, Pan YF, Yuan ZG, Chen DS, Song YJ, Gao Y. Immune-related RNA signature predicts outcome of PD-1 inhibitor-combined GEMCIS therapy in advanced intrahepatic cholangiocarcinoma. Front Immunol 2022;13:943066.
17. Yoshihara K, Shahmoradgoli M, Martínez E, et al. Inferring tumour purity and stromal and immune cell admixture from expression data. Nat Commun 2013;4:2612.
18. Yang Y, Wang Z, Fang J, et al. Efficacy and safety of sintilimab plus pemetrexed and platinum as first-line treatment for locally advanced or metastatic nonsquamous NSCLC: a randomized, double-blind, phase 3 study (oncology pRogram by innovENT anti-PD-1-11). J Thorac Oncol 2020;15:1636-46.
19. Yang Y, Sun J, Wang Z, et al. Updated overall survival data and predictive biomarkers of sintilimab plus pemetrexed and platinum as first-line treatment for locally advanced or metastatic nonsquamous NSCLC in the phase 3 ORIENT-11 study. J Thorac Oncol 2021;16:2109-20.
20. Davis AA, Patel VG. The role of PD-L1 expression as a predictive biomarker: an analysis of all US food and drug administration (FDA) approvals of immune checkpoint inhibitors. J Immunother Cancer 2019;7:278.
21. Paz-Ares L, Luft A, Vicente D, et al. KEYNOTE-407 Investigators. Pembrolizumab plus chemotherapy for squamous non-small-cell lung cancer. N Engl J Med 2018;379:2040-51.
22. Gadgeel S, Rodríguez-Abreu D, Speranza G, et al. Updated analysis from KEYNOTE-189: pembrolizumab or placebo plus pemetrexed and platinum for previously untreated metastatic nonsquamous non-small-cell lung cancer. J Clin Oncol 2020;38:1505-17.
23. Jeong HY, Kim H, Lee M, et al. Development of HER2-specific aptamer-drug conjugate for breast cancer therapy. Int J Mol Sci 2020;21:9764.
24. Xing X, Shi J, Jia Y, et al. Effect of neoadjuvant chemotherapy on the immune microenvironment in gastric cancer as determined by multiplex immunofluorescence and T cell receptor repertoire analysis. J Immunother Cancer 2022;10:e003984.
25. Cardoso F, van't Veer LJ, Bogaerts J, et al. MINDACT Investigators. 70-gene signature as an aid to treatment decisions in early-stage breast cancer. N Engl J Med 2016;375:717-29.
26. Pitt JM, Marabelle A, Eggermont A, Soria JC, Kroemer G, Zitvogel L. Targeting the tumor microenvironment: removing obstruction to anticancer immune responses and immunotherapy. Ann Oncol 2016;27:1482-92.
27. Hanoteau A, Newton JM, Krupar R, et al. Tumor microenvironment modulation enhances immunologic benefit of chemoradiotherapy. J Immunother Cancer 2019;7:10.
28. Kang Y, Huang J, Liu Y, Zhang N, Cheng Q, Zhang Y. Integrated analysis of immune infiltration features for cervical carcinoma and their associated immunotherapeutic responses. Front Cell Dev Biol 2021;9:573497.
29. Cieślik M, Chinnaiyan AM. Cancer transcriptome profiling at the juncture of clinical translation. Nat Rev Genet 2018;19:93-109.
30. Schakenraad A, Hashemi S, Twisk J, et al. The effect of tumor size and metastatic extent on the efficacy of first line pembrolizumab monotherapy in patients with high PD-L1 expressing advanced NSCLC tumors. Lung Cancer 2021;162:36-41.
31. Kim SI, Cassella CR, Byrne KT. Tumor burden and immunotherapy: impact on immune infiltration and therapeutic outcomes. Front Immunol 2020;11:629722.
32. Erbe R, Wang Z, Wu S, et al. Evaluating the impact of age on immune checkpoint therapy biomarkers. Cell Rep 2021;36:109599.
33. Kim J, Hurria A. Determining chemotherapy tolerance in older patients with cancer. J Natl Compr Canc Netw 2013;11:1494-502.
34. Conforti F, Pala L, Bagnardi V, et al. Sex-based heterogeneity in response to lung cancer immunotherapy: a systematic review and meta-analysis. J Natl Cancer Inst 2019;111:772-81.
35. Ye Y, Jing Y, Li L, et al. Sex-associated molecular differences for cancer immunotherapy. Nat Commun 2020;11:1779.
36. Ma J, Yao Y, Tian Y, Chen K, Liu B. Advances in sex disparities for cancer immunotherapy: unveiling the dilemma of Yin and Yang. Biol Sex Differ 2022;13:58.
37. Ulrich BC, Guibert N. Immunotherapy efficacy and gender: discovery in precision medicine. Transl Lung Cancer Res 2018;7:S211-3.
38. Atzpodien J, Wandert T, Reitz M. Age does not impair the efficacy of immunochemotherapy in patients with metastatic renal carcinoma. Crit Rev Oncol Hematol 2005;55:193-9.
39. Seymour L, Bogaerts J, Perrone A, et al. RECIST working group. iRECIST: guidelines for response criteria for use in trials testing immunotherapeutics. Lancet Oncol 2017;18:e143-52.
Cite This Article
Export citation file: BibTeX | RIS
OAE Style
Lin Z, Zhan J, Zhang L. ImmuneScore as a novel RNA-based prognostic signature superior to PD-L1 in advanced non-squamous NSCLC patients receiving chemotherapy combined with immune checkpoint inhibitor therapy. J Cancer Metastasis Treat 2024;10:11. http://dx.doi.org/10.20517/2394-4722.2023.139
AMA Style
Lin Z, Zhan J, Zhang L. ImmuneScore as a novel RNA-based prognostic signature superior to PD-L1 in advanced non-squamous NSCLC patients receiving chemotherapy combined with immune checkpoint inhibitor therapy. Journal of Cancer Metastasis and Treatment. 2024; 10: 11. http://dx.doi.org/10.20517/2394-4722.2023.139
Chicago/Turabian Style
Lin, Zhihuan, Jianhua Zhan, Li Zhang. 2024. "ImmuneScore as a novel RNA-based prognostic signature superior to PD-L1 in advanced non-squamous NSCLC patients receiving chemotherapy combined with immune checkpoint inhibitor therapy" Journal of Cancer Metastasis and Treatment. 10: 11. http://dx.doi.org/10.20517/2394-4722.2023.139
ACS Style
Lin, Z.; Zhan J.; Zhang L. ImmuneScore as a novel RNA-based prognostic signature superior to PD-L1 in advanced non-squamous NSCLC patients receiving chemotherapy combined with immune checkpoint inhibitor therapy. J. Cancer. Metastasis. Treat. 2024, 10, 11. http://dx.doi.org/10.20517/2394-4722.2023.139
About This Article
Special Issue
Copyright
Data & Comments
Data
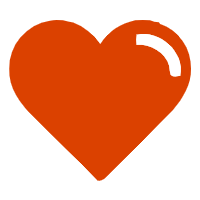

Comments
Comments must be written in English. Spam, offensive content, impersonation, and private information will not be permitted. If any comment is reported and identified as inappropriate content by OAE staff, the comment will be removed without notice. If you have any queries or need any help, please contact us at support@oaepublish.com.